Innovative tools offer reproducibility for Deep Learning
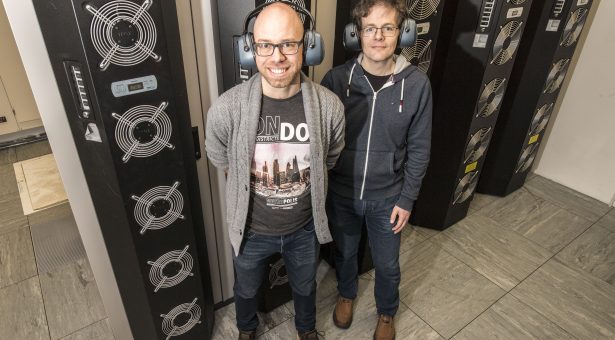
Machine Learning and AI are exciting topics in science, promising faster and more accurate data analyses and new insights.
However, they often create “black box” models that we can’t easily understand or reproduce. As science relies on our ability to build on previous work, this is dangerous for the future.
In a new study Dr Matthew Hartley and Dr Tjelvar Olsson from the Informatics team at the John Innes Centre show how we might be able to improve long term reproducibility for data analyses that rely on Deep Learning (DL) models.
DL techniques, a subset of machine learning, make use of artificial neural networks, simulated systems that mirror ways that real neurons work. Within biology DL has been applied to a wide range of problems such as cell image segmentation, genomic variant calling and transcription factor binding site prediction.
This paper provides both guidelines for reproducible model development, as well as a Python package, named dtoolAI which the team have developed.
“We’ve found these tools really useful in keeping our own AI models easier to understand and recreate, and we hope that others will too,” says Dr Hartley, head of Informatics at the John Innes Centre
The article ‘dtoolAI: Reproducibility for Deep Learning‘ appears in Pattern.