Artificial Intelligence works out the problem of seed germination tests
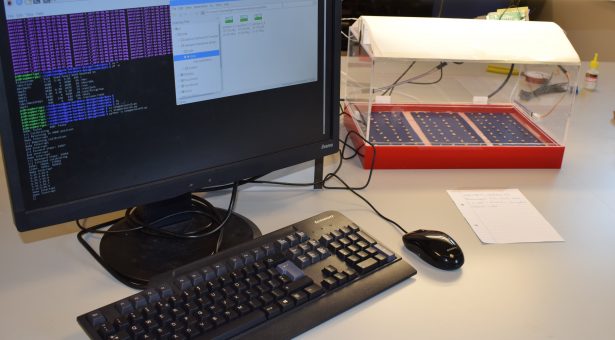
Researchers have taught a clever new tool how to carry out one of crop breeding’s more challenging tasks.
Growers need seeds that germinate effectively and uniformly within a given period to maximise crop productivity.
Seed suppliers must therefore test seed samples to ensure a certain germination rate is met, a process that is difficult and time consuming.
SeedGerm – based on machine learning-driven image analysis – performs this tricky process in a low-cost, high-throughput and semi-automated way.
The product is the result of a collaboration between the Earlham Institute (EI), the John Innes Centre, Syngenta and NIAB. Details of the research step-forward is published, open-access in New Phytologist, along with the open-source software and data.
Carmel O’Neill, Research Assistant in the Penfield Group at John Innes Centre and co-first author on the paper, said: “Currently most seed germination is still recorded manually. Against this, SeedGerm presents fast, accurate, high throughput screening and will be of major interest to crop seed production companies and research programs screening large germplasm collections.”
Machine learning – a version of artificial intelligence – involves teaching computer systems to interpret and translate vast quantities of data that are now available through increased processing power and cloud solutions.
SeedGerm uses a cabinet equipped with cameras that take photographs throughout the germination process, documenting each stage from imbibition (seeds taking up water) through to the emergence of the root and further changes in the newly growing plant.
Supervised machine learning is used to automatically determine how germination is progressing through comparing images. Algorithms can be trained to predict how likely it is that a seed has germinated based on measurements extracted from an image that relate to the seed’s size, shape, and colour.
Josh Colmer, a PhD student in the Anthony Hall Group at EI and co-first author on the paper, said: “To apply machine learning so effectively to test seed germination marks an exciting step forward, especially as the learnings from this project can inform a variety of image based analyses with wide-ranging applications in crop research.”
Seed germination experts from Syngenta confirmed the effectiveness of SeedGerm for measuring germination rate and seedling health across five major crop species, including tomato and rapeseed. It opens the way for SeedGerm replace manual seed scoring, while also contributing to seed certification, seed insurance and sowing guidance
In addition to being a quality assurance tool for seed companies and farmers, the power of SeedGerm to measure phenotypic changes over time has further novel applications in crop improvement research. Many of the characteristics that can be measured help to estimate performance in the field in terms of canopy closure, weed suppression and predicted yield.
Research at the John Innes Centre is already benefiting from the technology. The application of SeedGerm to a Genome Wide Association Study of rapeseed unearthed a chromosome region that explained the difference between low- and high-germinating seeds. Additionally, a gene in that region is related to one involved in abscisic acid (ABA) signalling in the model plant, which offers an interesting target for future studies and highlights the usefulness of SeedGerm in measuring the effect of genetic changes.
Dr Ji Zhou, formerly of EI and now Head of Data Science at NIAB, said: “We are excited to work jointly with Earlham Institute and the John Innes Centre to accomplish this seed germination research. It will provide next-generation solutions for both academic and industrial applications, including our NIAB LabTest services, so that we can supply impartial science-based advice and cost-effective help to seed testing and address related agricultural and horticultural challenges.”
“We are keen to continuously develop our Agri-Data and scientific computing capabilities together with both leading institutes, so that we can offer innovative solutions for the Agri-Food and the broader crop research community, strengthening the UK’s core competence in data science, Agri-Tech innovations, and AI-based crop informatics.”
Rene Benjamins, Senior Scientist at Syngenta Seeds said: “The developments and learnings from SeedGerm are truly a big step forward in automation and generating high quality and reliable data in scoring seed germination. This will help seed companies like Syngenta in providing the best quality to their customers.”
Read the paper ‘SeedGerm: a cost‐effective phenotyping platform for automated seed imaging and machine‐learning based phenotypic analysis of crop seed germination‘ in New Phytologist.
Josh Colmer is funded by UKRI-BBSRC under the Norwich Research Park Biosciences Doctoral Training Partnership (NRPDTP).