Life out there? Scanning plant space for new communication networks
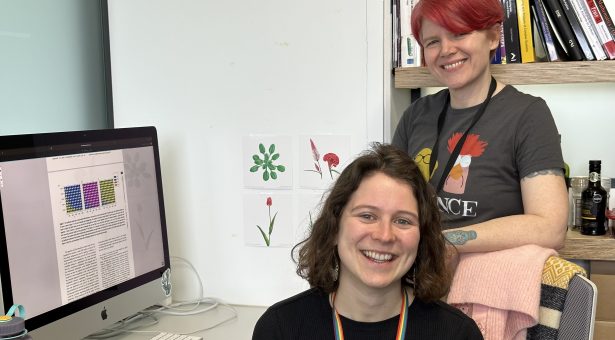
Postdoctoral researcher Franziska Hoerbst and Dr Melissa Tomkins are pioneering new computational-based techniques to track the root to shoot voyage of long-distance signalling molecules through plants.
Here the John Innes Centre researchers explain how in collaboration with German-based colleagues in PLAMORF they have made an important breakthrough in this fascinating area of research.
Communication and coordination are key for the existence of life. If we as humans want to work in a team to address a specific goal, we need to talk to each other.
The same kind of thing happens at cellular levels. All cells, from bacteria living in colonies, to billions of cells making up hundred-metre-high trees are talking to each other. If living cells want to work together, they need to communicate.
In humans, we send fast signals through the body in our nervous system to coordinate movement. Slower signals are sent through our hormonal system controlling hunger and other desires. These are communication systems that are based on the transport of signalling molecules.
We know less about this kind of signalling and communication in plants. But it has been shown that there are mobile molecules, even macromolecules like proteins and messenger RNAs (mRNA), that travel throughout the whole plant.
So, an mRNA produced in the root can leave the cell and go through the vasculature of the plant and can then be detected in a leaf.
This raised the question which we and our collaborators are trying to address: are macromolecules such as mobile RNAs part of a communication system?
A breakthrough came when it was shown that on a genomic scale high numbers of mobile RNAs were leaving the cell to travel from root to shoot, and back again through the plant vascular system.
Just as we scan space using detectors, scientists are also developing detection systems that zoom deeply into living organisms on earth.
That’s how we want to find out which plant communication systems there are and if some of them use macromolecules to convey messages about important developmental aspects such as nutrient availability or flowering time.
However, whether it’s scanning the sky or using computational methods to trace mobile RNAs, these detection systems both face the challenge of collecting a lot of “noise” – information that looks like what you are looking for but is in fact an error caused by the limitations of technology.
There is always the risk of interpreting something as a signal, which is a detection error.
This problem is highlighted in the process of tracking plant mobile RNAs using modern sequencing techniques.
To trace RNAs going from one part of the plant to another, researchers in the PLAMORF collaboration use a technique that involves grafting together two plants which are genetically almost identical, apart from some point mutations.
Grafting is a way of shedding light on long distance signalling in plants because it allows researchers to trace RNAs leaving the cells from one plant travelling from the root, across the graft to the shoot in the other plant.
However, for this technique to work, researchers must be able to distinguish between a mutation in a mobile RNA and what might be an error in the computational sequence readout.
Sequencing techniques, while incredibly precise are not error free, so in the past plant researchers did not know if they were looking at a sequencing error or a signal for a long-distance mobile RNA?
This was the nature of the problem addressed in our study which appeared in Royal Society Interface in which we developed a novel statistical model to help differentiate errors from signals in the detection of travelling macromolecules in plants.
The new method uses the power of Bayesian statistics (a branch of statistics) as part of a software package that examines datasets collected for mobile RNA detection. Researchers then get a probability of whether what they are looking at is noise or a mobile RNA.
This method of filtering out statistical errors exhibited near faultless performance on simulated datasets over a wide range of parameters as well as controlled experiments using real data.
The study reveals that a handful of carefully derived equations can drastically improve the accuracy of a detector. The results are stunning! We believe the same methodology will find wide applications in other areas where detecting signals where noisy data is a challenge.
The development of this new method was a true team effort between experimental biologists and computational scientists who are now able to explore their research questions with better data than ever before.
Great things can happen if communication works – that’s another conclusion of our study.
PLAMORF is an international research collaboration between the John Innes Centre in the UK, the Max Planck Institute for Plant Physiology and the University of Hamburg, both in Germany, was awarded an ERC (European Research Council) Synergy grant.